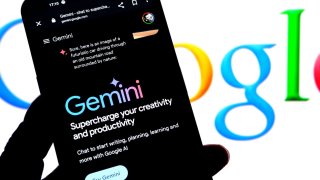
- When Google recently took its Gemini image-generation feature offline for further testing because of bias issues, the episode raised red flags about the potential dangers of generative artificial intelligence.
- Depending on the data that gen AI is trained on, the model learns and reflects that in its outputs.
- Ensuring transparency in how generative AI systems operate and make decisions is crucial for building trust and addressing bias concerns.
When Google took its Gemini image-generation feature offline last month for further testing because of issues related to bias, it raised red flags about the potential dangers of generative artificial intelligence, not just the positive changes the technology promises to usher in.
"Companies need to overcome bias if they wish to maximize the true potential of this powerful technology," said Siva Ganesan, head of the AI Cloud business unit at Tata Consultancy Services. "However, depending on the data that Gen AI is trained on, the model learns and reflects that in its outputs," he said.
Crucial to managing issues of potential bias in AI is to have clear processes in place and prioritize responsible AI from the beginning, said Joe Atkinson, chief products and technology officer at consulting firm PwC.
Get Tri-state area news delivered to your inbox.> Sign up for NBC New York's News Headlines newsletter.
"This starts with striving to make gen AI systems transparent and explainable, giving users access to clear explanations of how the AI system makes decisions and being able to trace the reasoning behind those decisions," Atkinson said.
Ensuring transparency in how generative AI systems operate and make decisions is crucial for building trust and addressing bias concerns, said Ritu Jyoti, group vice president, AI and automation, market research and advisory services at International Data Corp.
"Organizations should invest in developing explainable AI techniques that enable users to understand the reasoning behind the AI-generated content," Jyoti said. "For example, a healthcare chatbot powered by generative AI can provide explanations for its diagnoses and treatment recommendations, helping patients understand the underlying factors and mitigating potential biases in medical advice."
Money Report
Diversity in AI development teams, data
Companies also need to create diverse and inclusive development teams. Including people who represent a range of backgrounds, perspectives, and experiences, "goes a long way in identifying and mitigating biases that may inadvertently be embedded in the AI system," Atkinson said. "Different viewpoints can challenge assumptions and biases, leading to fairer and more inclusive AI models."
Another good practice is to build robust data collection and evaluation processes.
"We've seen companies who are eager to start with AI models without first addressing the existing underlying data," Ganesan said. "By pulling in diverse, representative data sets, organizations can mitigate biases. Organizations should track data changes and distribution to enhance AI model development and ensure explainability."Ā
Biases can arise if the training data is limited or skewed towards certain demographics, Atkinson said. "By collecting data from a wide range of sources and making sure it is representative of the population, companies can reduce the risk of biased outcomes," he said.
Using diverse and representative datasets "is crucial to ensure that the data used for training generative AI models is free from discriminatory patterns and accurately reflects the diversity of the intended user base," Jyoti said.
For instance, when developing a language generation model for customer service interactions, the training data should include a range of customer profiles to avoid biased responses that favor certain demographics, Jyoti said.
Continuous evaluation of an AI system's performance is also important to help identify and rectify any biases that may arise.
"Regularly monitoring the outputs of generative AI systems is essential to identify and mitigate biases," Jyoti said. "Organizations should establish evaluation frameworks and metrics to assess the fairness and ethical implications of the generated content. For example, a news organization employing a generative AI model to produce news articles can analyze the articles for biased language or perspectives and make necessary adjustments to ensure balanced and unbiased reporting."
Keeping humans in the loop
It's also vital to keep humans in the loop and provide upskilling opportunities for people looking to develop gen AI tools. "It's important for leaders to provide training and awareness when it comes to responsible AI use," Atkinson said. "This includes fostering a culture of responsible AI use by educating them on potential risks, encouraging cautious usage of AI-generated content, and emphasizing the need for human review and verification."
Incorporating human reviewers or moderators in the generative AI pipeline can help mitigate risks, Jyoti said. "Human intervention can provide a checks-and-balance system to prevent the propagation of biased or harmful content," she said.
For example, social media platforms using generative AI for content recommendation can employ human moderators to review and filter out potentially biased or inappropriate content, ensuring a safer and more inclusive online environment.
In addition, companies should set up systems for gathering input and feedback. "Creating channels for users to report inaccuracies or unexpected outputs is critical to knowledge sharing and making sure you are catching inconsistencies or biases before it becomes a widespread problem," Atkinson said.
From an overall industry maturity perspective, collaborative efforts and industry standards will be crucial, Jyoti said.
Sharing knowledge, experiences, and tools can accelerate progress in addressing bias and improving the overall ethical use of generative AI, she said. "For instance, AI conferences and industry associations can facilitate discussions and knowledge exchange on bias mitigation techniques and ethical considerations in generative AI applications," she said.
While the Gen AI market is nascent and rapidly evolving, and while some of the problems are complex, more due diligence in the training and tuning of models is needed, Jyoti said. "The stakes are high," she said.
Due to the fast pace of gen AI innovation, any bumps along the road need to beĀ handled swiftly, Ganesan said, and it's good that Google responded quickly to the bias problem.Ā "Much of the heavy lifting that is required behind the scenes can help companies get things right,Ā and that can improve outcomes," he said.